Hoffmann Research Group
Computational Biology of Aging:
The Algorithm of Growing Old
The Computational Biology Group is a young research team. One of our primary interests is to contribute to a better understanding of the epigenetic control of transcription. To do this, we are developing methods for the analysis of big and multidimensional biological data sets. Our work is highly collaborative and we are and have been a member of several high-profile national and international consortia such as the International Cancer Genome Consortium (ICGC) or the BLUEPRINT Consortium.
Below we have highlighted some of our research interests.
Research Interests
The integration of epigenetic information including DNA-methylation and histone modification data with transcriptomics, provides new insights into the mechanisms of epigenetic control.
As a part of the German ICGC-MMMLSeq and ICGC-DE-mining consortia as well as the HNPCCSys and BLUEPRINT consortia, we help to analyze hundreds of datasets from various tumor types. Our goal is the identification of common epigenomic mechanisms shared by many tissues, e.g. differentially methylated regions (DMRs).
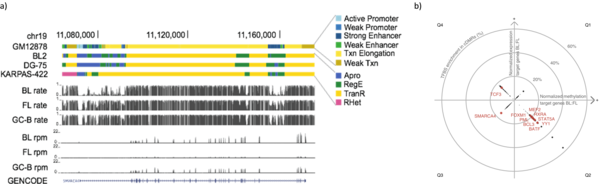
Methylated DNA is one of the main epigenetic modifications. We implemented the functionality to map bisulfite sequencing derived reads (Otto et al., 2013) into our alignment software Segemehl (Hoffmann et al., 2009). This enables easy translation of read numbers into methylation levels.
To identify significantly different methylation levels between certain conditions and among larger numbers of samples, we developed a program called metilene (Juehling et al., 2016). This software is able to rapidly identify differentially methylated regions (DMRs) with high confidence.
In RNA-Sequencing, reads originate from mRNAs or ncRNAs and can span exon junctions. The biological process leading to exon junctions is called splicing. We have extended our alignment software Segemehl with an algorithm to handle and to report splicing events. To detect alternative splicing events that play a major role in differentiation and diseases, we developed the software DIEGO (Doose et al., 2017). The results of DIEGO have been crucial for a number of our publications on lymphoma.
Recently, we analyzed bivalent chromatin states in cancer. This bivalent (poised or paused) chromatin comprises activating and repressing histone modifications at the same location i.e. promoter or enhancer regions. Specific combinations of epigenetic marks keep gene expression low but poise genes for rapid activation. Typically, DNA at bivalent promoters is lowly methylated in normal cells, but frequently show elevated methylation levels in cancer samples. We were able to propose a universal classifier built from chromatin data that is able to identify cancer samples solely from hypermethylation of bivalent chromatin. Tested on more than 7,000 DNA methylation data sets from several cancer types, it reaches an area under the curve (AUC) of 0.92. Although higher levels of DNA methylation are often associated with transcriptional silencing, counter-intuitive positive statistical dependencies between DNA methylation and expression levels have been reported previously. The re-analysis of combined expression and DNA methylation data sets from over 5,000 samples demonstrated the surprising coincidence that hypermethylation of bivalent chromatin and up-regulation of the corresponding genes is a general phenomenon in cancer. Such events affect many developmental genes and transcription factors, including dozens of homeobox genes and other genes implicated in the transcriptional landscape of cancer cells. Thus, we conclude that the disturbance of bivalent chromatin may be intimately linked to tumorigenesis.
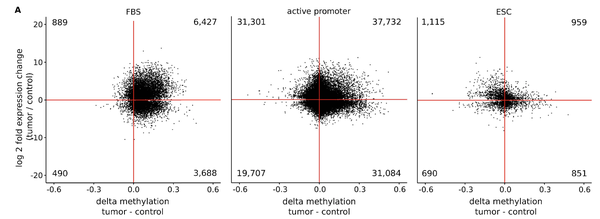
Contact
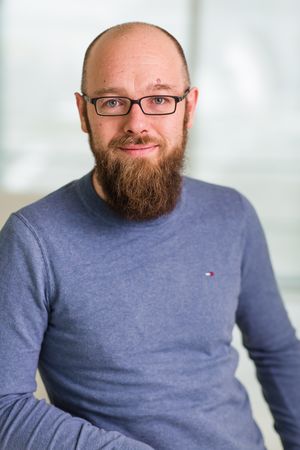
Steve Hoffmann
Group Leader
+49 3641 656810
steve.hoffmann@~@leibniz-fli.de
Patricia Möckel
Assistance
+49 3641 65-6240
patricia.moeckel@~@leibniz-fli.de